A South Korean research team has developed a quantum artificial intelligence (AI) algorithm that surpasses current AI technologies. It is expected that this new algorithm can be used for “nonlinear machine learning” that has been difficult to implement within the quantum AI field.
Korea Advanced Institute of Science and Technology (KAIST, President Shin Sung-chul) made an announcement on July 7th that a research team led by Professor Rhee June-koo (Director of KAIST ITRC (Information Technology Research Center) of Quantum Computing for AI center) of KAIST School of Electrical Engineering along with a research team led by Germany and South Africa co-developed a nonlinear quantum machine learning AI algorithm.
Quantum AI is AI that can be used for quantum computers. Because how a quantum computer operates is completely different from how a normal computer operates, development of new quantum algorithms has been needed desperately.
The key is to materialize nonlinear (high order) machine learning. If linear machine learning involves linear equations, nonlinear machine learning involves quadratic and cubic equations resulting in more complicated machine learning.
“Data feature classification”, which is absolutely necessary for machine learning, becomes much easier when machine learning is not linear. Due to a higher dimension of “information space” that is utilized for nonlinear machine learning, classification of features can be done in more detail.
Although features may look clustered in a 2D graph, they are actually separated when they are viewed within a 3D space. As a result, one can find new differences in features that could not be seen from a 2D dimension.
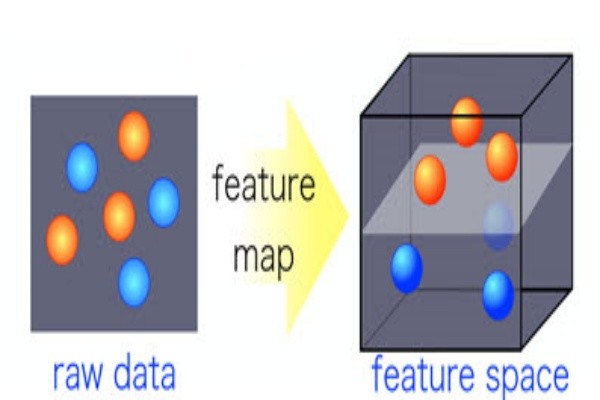
However, “nonlinear kernel technology” is needed in order to observe new differences in features. Kernel is a function that quantifies similarities between data that is used for machine learning and it is a core foundation for nonlinear machine learning.
If nonlinear machine learning is applied to characteristics of quantum computing, they can create enormous amount of synergy. Because quantum computing is good for parallel computation and is proportional to number of qubits (basic unit of quantum information in quantum computing), a dimension of information space increases. Operation is possible even with a small amount of computation.
The research team led by Professor Lee designed a nonlinear kernel during its research and developed a quantum machine learning algorithm that can perform nonlinear machine learning. It has created a quantum algorithm system through the quantum forking technology that processes data as quantum information and enables parallel operation and a simple quantum measurement technology. It also demonstrated its ability to materialize various quantum kernels.
The research team utilized IBM’s qubit cloud service and proved its quantum AI algorithm and its performance through demonstrations.
“Kernel-based quantum machine learning algorithm will surpass outdated kernel-based supervised learning.” said Park Kyung-deok who is a research professor at KAIST School of Electrical Engineering and participated in the research.
Staff Reporter Kim, Youngjun | kyj85@etnews.com